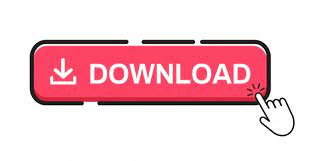
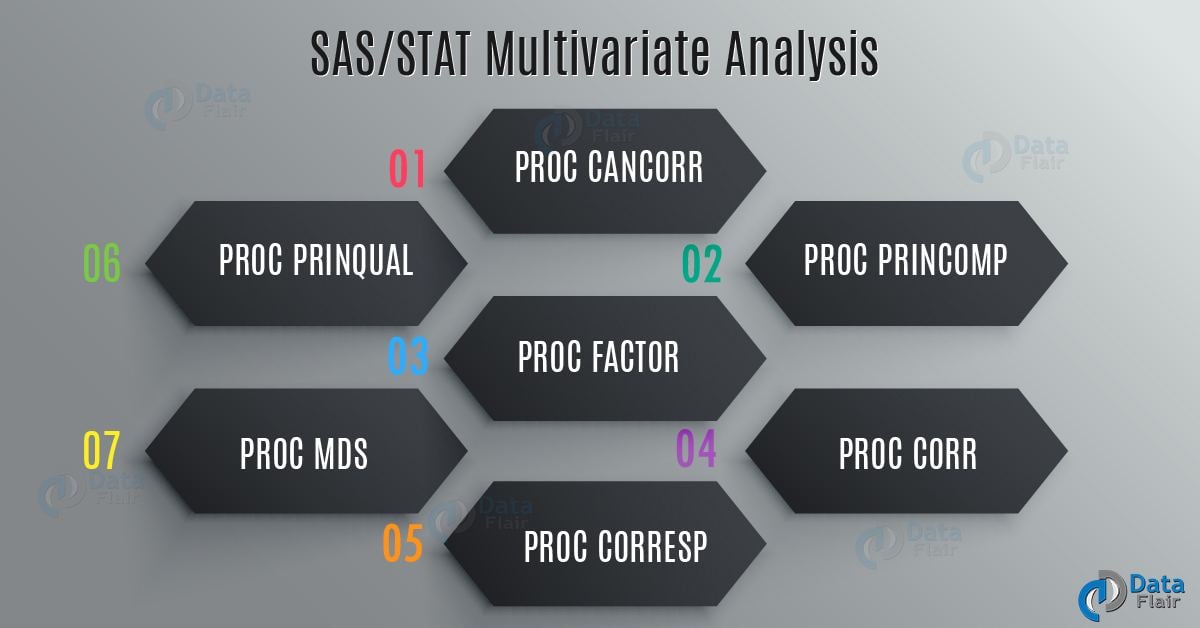
To facilitate exposition of the method of estimation by simulated maximum likelihood, let us focus on the case in which M = 3.
Sas jmp multivariate tutorial free#
That is, the multivariate probit model can be used to fit a univariate probit model for panel (cross-sectional time-series) data allowing for a free correlation structure over time. Alternatively, the y im might represent M outcomes on the same choice at M different points in time. The y im might represent outcomes for M different choices at the same point in time, for example, whether an individual owns each of M different consumer durables. The familiar univariate and bivariate probit models correspond to the cases when M = 1 and 2 (estimable using probit and biprobit). As for the SUR case (sureg), the equations need not include exactly the same set of explanatory variables. The model has a structure similar to that of a seemingly unrelated regression (SUR) model, except that the dependent variables are binary indicators.
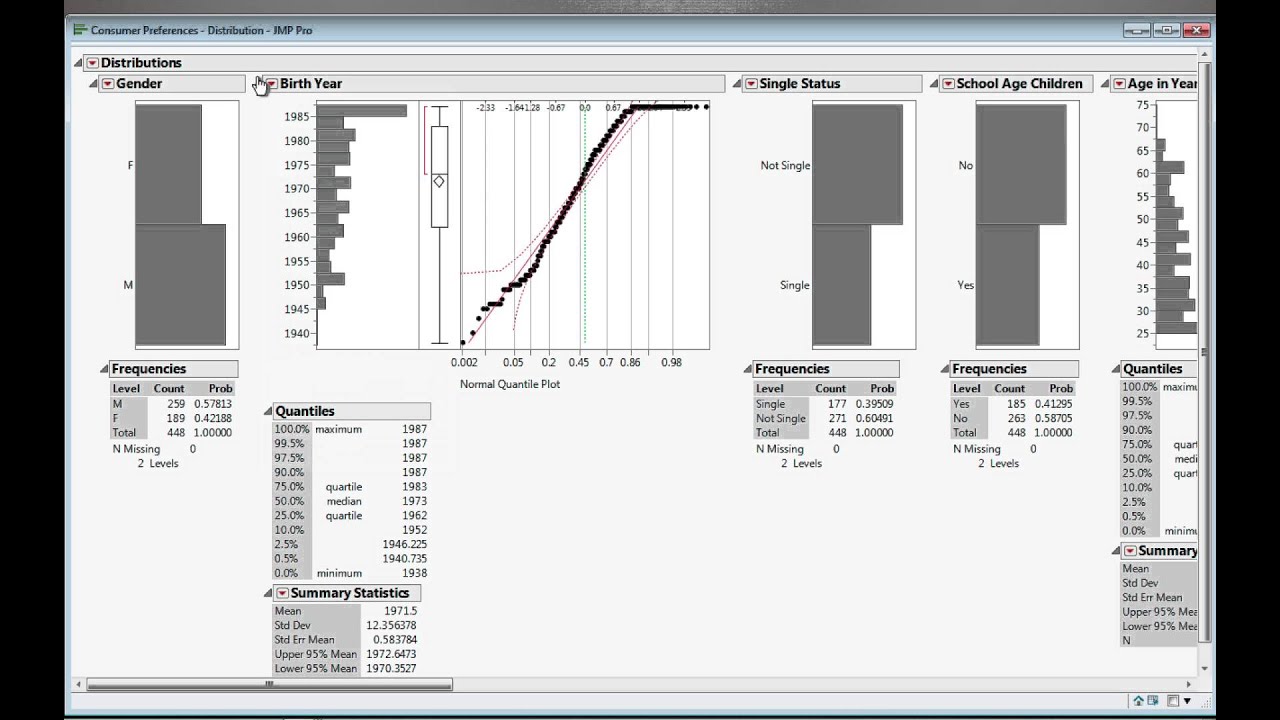
Jenkins The model and the method of simulated maximum likelihood Consider the M-equation multivariate probit model: y im = β m X im + ǫ im,m=1.,m y im =1ify im > 0 and 0 otherwise ǫ im,m=1.,m are error terms distributed as multivariate normal, each with a mean of zero, and variance covariance matrix V, where V has values of 1 on the leading diagonal and correlations ρ jk = ρ kj as off-diagonal elements. In mvprobit, written independently, a more general algorithm is used, the number of model equations is unlimited in principle, there are more options, and there is also a companion postestimation prediction program (mvppred). Antoine Terracol s program triprobit, available from the SSC-IDEAS archive, fits trivariate probit regression models using simulated likelihood estimation. Section 5 discusses issues such as the choice of number of replications. Our mvprobit program is explained in section 3, and it is illustrated in section 4. In section 2, we describe the model and review the principles underlying estimation by simulated maximum likelihood using the so-called GHK simulator. In this article, we discuss the application of a simulation method to maximum likelihood estimation of the multivariate probit regression model and describe a Stata program mvprobit for this purpose. Greene (2003, ) provides a brief textbook exposition. See Stern (1997) and Gourieroux and Monfont (1996) for extensive discussion of simulation estimation techniques and their applications in a number of contexts. Researchers have turned instead to simulation-based methods that have much better properties. Moreover, in these multivariate normal cases, computations based on standard linear numerical approximations, such as those based on the Newton Raphson method, are relatively inefficient and may provide poor approximations (Hajivassiliou and Ruud 1994). (See, for example, norm and binorm in Stata 8.) Accurate functions for the evaluation of trivariate and higher-dimensional normal distributions do not exist in Stata, however.
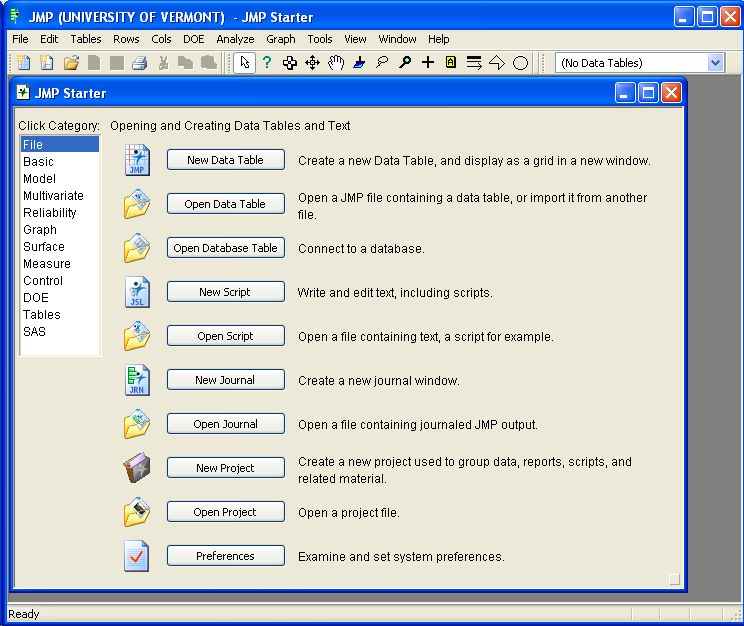
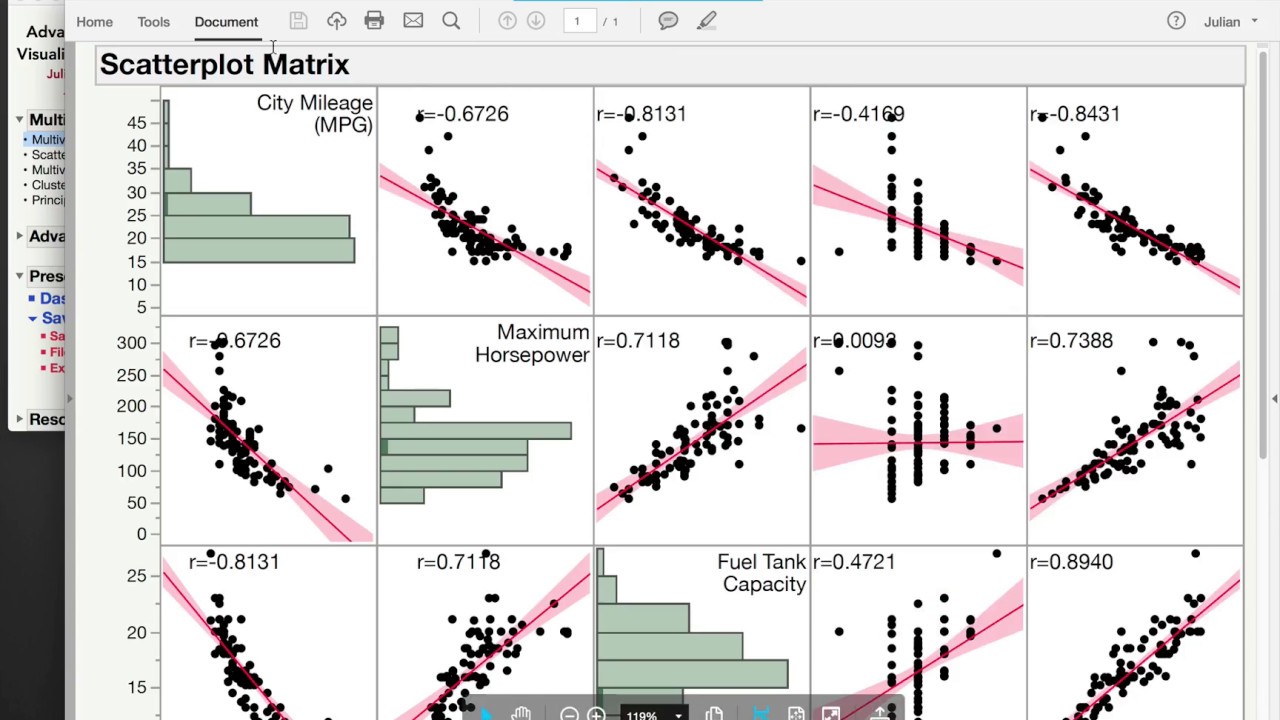
Sas jmp multivariate tutorial software#
Algorithms exist that provide accurate calculations for univariate and bivariate normal pdfs, and these are used by functions incorporated in many software packages. Keywords: st0045, maximum likelihood estimation, multivariate probit regression model, GHK, mvprobit, mvppred 1 Introduction Evaluation of probit-model likelihood functions requires calculation of normal probability distribution functions. We discuss the application of the GHK simulation method for maximum likelihood estimation of the multivariate probit regression model and describe and illustrate a Stata program mvprobit for this purpose. 1 The Stata Journal (2003) 3, Number 3, pp Multivariate probit regression using simulated maximum likelihood Lorenzo Cappellari Università del Piemonte Orientale and University of Essex Stephen P.
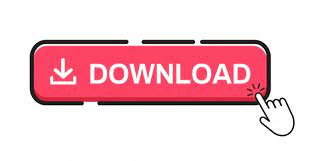